Research Grants
- Principal Investigator – Development of a novel cloud-based digital health system for real-time prediction of heartrate from smartwatch, NSERC Alliance Grant, 2024-2026, $147,567.
- Principal Investigator – Development of a Compassionate Digital Health System to Support Patients with Dementia and their Caregivers in the Community, with a Focus on Inclusion and Caregiver Support in Home Deployment, AMS Small Grant in Compassion and Artificial Intelligence, 2023-2024, $30,000.
- Principal Investigator – Predicting Future Anomalous Events, Data Science Institute Seed Grant, University of Toronto, 2023-2024, $10,000.
- Co-pplicant – Digital Ageism and Smart Home Technologies to Care for Older Adults, CIHR Institute of Aging Planning and Dissemination Grant - Institute Community Support, 2024, $47,655.
- Principal Investigator – Assessing exercise quality from videos with deep learning approaches, Transform-HF Undergraduate Research Program, 2023, $6,000.
- Principal Investigator – Predicting the onset of responsive behaviors among patients living with dementia in the community, Longitude Prize on Dementia Discovery Award (23/175 applicants), 2023-2024, £80,000 (≈ $135,608).
- Co-Principal Investigator – Multimodal AI-based Sensor platform for Older Individuals (MAISON), Spark+ Program, CABHI, 2023, $75,000.
- Co-Principal Investigator – A Multimodal Toolkit for Assessing Frailty at Home, Spark Program, CABHI, 2023, $50,000.
- Co-Principal Investigator – Co-designing a multi-modal sensor system to enable person-centered hip-fracture recovery at home for older adults, EPIC-AT Early Career Researcher Awards in Patient-Oriented Research, AGE-WELL NCE, 2023, $20,000.
- Co-Principal Investigator – Multisensor technology to safely navigate exercise in extreme temperatures, MITACS and Vee Technologies, 2023-2025, $189,000.
- Principal Investigator – Development of AI framework for the prediction of future anomalous events, NSERC Alliance International Catalyst Grant, 2023, $25,000.
- Principal Investigator – Pilot Study to Predict Social Isolation in Older Adults Living in the Community with a Novel Cloud-based Digital Health Platform, AGEWELL ECR Grant Program, 2022, $7,500.
- Principal Investigator – Inclusion of Older Adults with Dementia and their Caregivers in Deploying Multimodal Sensors in their Homes., EPIC-AT Early Career Researcher Patient Engagement Supplement, 2022, $5,000.
- Co-Principal Investigator – Exploring ethical and societal impact on smart home-based elderly care from a cross cultural and interdisciplinary perspective. University of Toronto and Zhejiang University Seed Grant, 2022, $20,000.
- Principal Investigator – AVA: Adaptive Virtual Rehabilitation Assistant Powered by Artificial Intelligence, NFRF Pandemic Response, 2022, $237,814.
- Principal Investigator – Detecting Behaviours of Risk in Nursing Homes using Deep Learning, Alzheimer’s Association Research Grant, 2022, US $149,991 / C$187,489.
- Co-Investigator – Early Professionals, Inspired Careers in AgeTech (EPIC-AT): Innovators of Tomorrow Health Research Training Platform in AgeTech, Training Grant: Health Research Training Platform, $2,399,754.
- Co-Principal Investigator, Second Indo-Canadian Conference on Artificial Intelligence and Rehabilitation Robotics, Shastri Indo-Canadian Institute: Conference and Lecture Series Grant, with Drs. Alex Mihailidis and Dinesh Jayagopi, 2022, INR 50,000.
- Principal Investigator – Artificial Intelligence driven Personalized Virtual Cardiac Rehabilitation, J.P. Bickell Foundation, Medical Research Grant, 2022, $78,740.
- Principal Investigator – LG Electronics AI Specialist Program, 2021, $24,000.
- Principal Investigator – Examining the paradox of smart homes under the contemporary lens of surveillance theory, SSHRC Insight Grant, 2021-2023, $86,614.
- Co-Investigator – Artificial Intelligence based adaptive and interpretable models for analyzing multi-track epigenomic sequential data, CIHR Team Grant: Canada-Japan CEEHRC Teams in Advancing Epigenetic Technologies, Dr. Ahmed Ashraf (PI) and Dr. James Davie (Co-I), 2021-2023, $298,500.
- Co-Principal Investigator, Shastri Indo-Canadian Institute's Golden Jubilee Conference and Lecture Series Grant to conduct the Indo-Canadian Conference on Artificial Intelligence and Rehabilitation Robotics with Dr. Alex Mihailidis and Dr. Abhinav Dhall, 2021, INR 110,000.
- Principal Investigator – Development of an Artificial Intelligence driven Personalized Home-based Cardiac Telerehabilitation platform to determine Patient Engagement in their respective Cardiac Rehabilitation program, AMS Small Grant in Compassion and Artificial Intelligence, Dr. Tracey Colella (Co-PI), 2021, $19,975.
- Co-Principal Investigator – Development of machine learning and deep learning algorithms for imbalanced and one-class classification problems (PI - Dr. Amir Ahmad), United Arab Emirates University Strategic Research Program, 2021-2024, Overall Award (AED 740,000), Co-PIs Award $34,590 (AED 100,000).
- Co-Applicant – Ageism in A.I.: Exploring the Social and Ethical Implications of Age-Based Bias in Artificially Intelligent Systems (PI - Dr. Charlene Chu, SSHRC Insight Development Grant, 2020-2022, $63,059.
- Principal Investigator – Location tracking-based health status indices and their integration into clinical support tools in long-term care, AGEWELL NCE, Core Research Program Awards, 2020-23, $573,980.
- Principal Investigator – Unsupervised Deep Learning Framework for Solving One Class Classification Problems, NSERC Discovery Grant, 2020-2025, $132,500.
- Nominated Principal Applicant – Development of Machine Learning Models to Characterize Homebound Status in Older Adults in Canada, (PA - Andrea Iaboni), CIHR Catalyst Grant : Analysis of CLSA Data, 2020-22, $60,000.
- Principal Applicant – Using in-home sensors to detect the physiological, functional, and behavourial changes related to ingesting cannabidiol oil in older adults with dementia living at home (Nominated Principal Applicant - Dr. Charlene Chu), CIHR SPOR, 2020-22, $90,300.
- Co-PI – Developing an at-home sensor system to detect social isolation and functional decline in high-risk older adults in the community (PI - Dr. Charlene Chu), CABHI SPARK, 2019-20, $49,961.
- Co-PI – Artificial Intelligence for the detection of anomalous events in long term care (PI - Andrea Iaboni), CABHI SPARK, 2019-2020, $49,972.
- PI – Location tracking-based health status indices and their integration into clinical support tools in long-term care., (PI - Andrea Iaboni), AGEWELL NCE Catalyst CRP Funding, 2019-2020, $30,000.
- Co-Applicant – Intelligent intervention system to deter older people (living with mild dementia) from physical inactivity, (PI - Kristine Newman), Ryerson FCS Seed Grant, 2018-19, $6,000.
- Assessing and predicting the physiological, functional, and behavourial effects of oral cannabidiol oil in older adults with dementia living at home using in-home sensors: a pilot study, Alzheimer’s Society Research Program, New Investigator Grant, Dr. Charlene Chu (PI), 2021-2023, $200,000.
- CareBand: Wearable technology for people with dementia, (PI - Adam Sobol), NIH SBIR Grant, 2019-2020, US $228,778
Major Research Projects
Virtual Rehabilitation (VR) or Telerehabilitation aims to reduce the duration of hospitalization and the costs to patients and health care providers by transferring patient care to the home following the acute phase of disease. VR is especially beneficial for patients who may live far from rehabilitation centers or in remote areas where traditional rehabilitations services may not be easily accessed. Despite the benefits of VR, there is a high rate of patient dropout due to several barriers, including lack of confidence or motivation, scheduling conflicts, caregiver responsibilities, geographical location, transportation, and financial constraints. In this project, we are building an Artificial Intelligence driven Avatar system to detect patient engagement, assess exercise quality and predict their dropout risks in the program.
Providing care for a rapidly aging population, especially people living with dementia (PLwD), constitutes a major challenge for global healthcare. Older adults with advanced dementia living in long-term settings often have behavioural and psychological symptoms of dementia, with agitation and aggression amongst the most common symptoms. These behaviours can lead to resident-on-resident violence and workplace violence towards staff in long-term care facilities. In this project, our goal is to develop an automated system that can alert staff and provide personalized interventions when episodes of agitation occur by detecting, tracking over time, and predicting such behaviours among PLwD. We are using a multi-modal sensor network that collects data using physiological and motor sensors, video cameras, motion/door sensors and pressure mats to build novel machine/deep learning classifiers.

A fall is an abnormal activity that occurs rarely, infrequently and diversely. Therefore, it is difficult to collect training data for falls. Traditional machine learning classifiers may not work well in such skewed scenario. We are taking an alternative approach to only train classifiers on normal activities that are present in abundance and flag a fall as an abnormal activity. We are using vision based non-invasive sensors that protect privacy, such as thermal camera, depth camera and near-IR camera to test our models. We are developing models using 3D Spatio-Temporal Convolutional Autoencoders (3D-STCAE) to achieve this task. A short demo to detect an unseen fall using 3D-STCAE is shown below.
- Received Ryerson's FCS Seed Grant with Dr. Kristine Newman
- Received CABHI' Spark Grant with Dr. Charlene Chu
An Intelligent Assessment system is being developed to detect Social Isolation, Functional and Cognitive decline among older adults. This system is a zero-effort assistive technology solution that utilizes low cost smart devices in a home setting and requires little or no effort from the people using it. The system will detect prolonged periods of inactivity, motion and other physiological and ambient indicators suitable for the task. The goal of this system is to develop a passive assistive system that does not aggressively prompts the person using it, rather adaptively learns their daily behaviour. A survey on the role of technology for detecting social isolation is currently being developed.
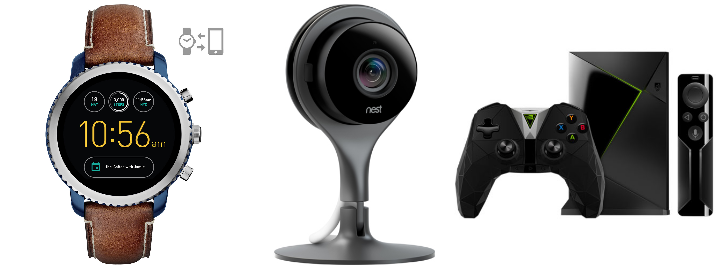
Team Members
- Khai De Luc, MASc student, 2024 -
- Anshul Verma, PhD Student, 2023 -
- Jahir Ibna Rafiq, MASc Student, 2023 -
- Zain Hasan, PhD Student, 2023 -
- Fateme Pourghasem, PhD Student, 2023 -
- Sadaf Safa, MASc Student, 2022 -
- Yasser Karam, MASc student, 2022 -
- Faranak Dayyani, MASc student, 2022 -
- Faezah Zahari, PhD student, (with Dr. Milos Popovic), 2021 -
- Pratik Mishra, PhD student, 2021 -
- Reza Basiri, PhD Student (with Dr. Milos Popovic), 2019 -
- Ali Abedi, Sr. Sc. Associate, October 2023 -
- Smit Patel, Research Analyst, 2024 -
- Grant Lau, Undergraduate Researcher, 2023
- Ryan Zhang, Undergraduate Researcher, 2023
- Shivam Bhat, Volunteer Researcher, 2023
- Zara Hasan, Clinical Researcher, 2023 -
- Ahmed Mokhtar, Undergraduate Researcher, 2023
- Tianyu Shi, Graduate Researcher, 2023
- Ruile Lu, Work Study Student, Undergraduate Researcher, 2023
- Ali Abedi, Postdoctoral Fellow, October 2020 - September 2023
- Chau Nguyen, Work Study Student, 2023 - 2024
- Shlok Desai, Work Study Student, 2023 - 2024
- Sahail Barakzi, Work Study Student, 2023 - 2024
- Canyang Wang, Work Study Student, 2023 - 2024
- Darcy Wang, Work Study Student, 2023 - 2024
- Smit Patel, Undergraduate Researcher, 2022 - 2023
- Jessica P. Campbell, Clinical Research Assistant, 2021 - 2023
- Chengshuo Zhang, Undergraduate Researcher, 2023
- Aliana Jamal, Undergraduate Researcher, 2023
- Mark Karlov, Undergraduate Researcher, 2023
- Swati Katyarmal, Volunteer Researcher, 2023
- Batuhan Karagoz, Undergraduate Researcher, 2022 - 2023
- Azra Ince, Research Assistant, 2023
- Tun Zheng, Clinical Researcher, 2023
- Elham Khodabandehloo, Postdoctoral Researcher, January 2022 - April 2023
- Jacob Buchan, Work Study Student, 2021 - 2023
- Tamim Faruk, Research Assistant, 2023
- Mobin Malmirian, Undergraduate Researcher, 2022 - 2023
- Shirley Cui, Associate Technical Analyst, 2020 - 2023
- Abeer Badawai, Visiting PhD student, 2022 - 2023
- Zhidong Meng, Visiting PhD student, 2022
- Stefan Denkovski, MASc Student, (with Dr. Alex Mihailidis), September 2019 - 2022
- Tamim Faruk, MASc student, 2020 - 2022
- Zakary Georgis-Yap, MASc student, 2020 - 2022
- Alina Pirani, Co-op student, 2022
- Harry Parmar, Undergraduate Trainee, 2022
- Alicia Natividad, Work Study Student, 2021 - 2022
- Yanchen Ma, Undergraduate Thesis Student, Engineering Science, 2021-2022
- Clarisse Misola, Undergraduate Thesis Student, Psychology and Health, 2021-2022
- Isaac Chang, MASc student, 2021
- Sally Moon, MASc Student, (with Dr. Alex Mihailidis), September 2019 - January 2022.
- Logan Rooks, Associate Technical Analyst, 2020 - 2021.
- Komal Saini, Undergraduate Researcher, 2021.
- Sofija Spasojevic, Postdoctoral Researcher, Primary Supervisor, September 2018- August 2020, Co-supervisor with Dr. Alex Mihailidis, September 2020-August 2021.
- Clara Guo, Undergraduate Thesis Student, Engineering Science, 2020-2021.
- Maruti Patel, Undergaduate Researcher Intern, 2020.
- Mohammadreza (Reza) Faieghi, Postdoctoral Researcher (with Dr. Andrea Iaboni), March 2019 - July 2020.
- Nizwa Javed, Research Analyst, 2020.
- Katherine Rich, Undergraduate Student, 2020.
- Sepehr Rashidi, Volunteer Researcher, 2020
- Logan Rooks, Undergraduate Thesis Student, Engineering Science, 2019-2020
- Matthew Nogas, Technical Analyst (2018-2019), Undergraduate Trainee (2018)
- Thaejaesh Sooriyakumaran, Undergraduate Summer Intern, 2019
- Brandon Malamis, Undergraduate Summer Intern, 2018, 2019
- Paris Rosarie, Undergraduate Summer Intern, 2019
- Jacob Nogas, Undergraduate student (PEY year), 2017-2018
- Tong (Maggie) Zhu, Undergraduate Summer Intern, 2017